1. What type of magnetic material is used in making permanent magnets? [1]
2. Which physical quantity has the unit wb/m2
? Is it a scalar or a vector quantity? [1]
3. Define angle of dip. Deduce the relation connecting angle of dip and horizontal
component of earth’s total magnetic field with the horizontal direction.
[2]
4. A point change +q is moving with speed υ
perpendicular to the magnetic field B as shown
in the figure. What should be the magnitude and
direction of the applied electric field so that the
net force acting on the charge is zero?
[2]
5. The energy of a charged particle moving in a uniform magnetic field does not
change. Why?
[2]
6. In the figure, straight wire AB is fixed; white the
loop is free to move under the influence of the
electric currents flowing in them. In which
direction does the loop begin to move? Justify.
[2]
7. State two factors by which voltage sensitivity of a moving coil galvanometer
can be increased?
[2]
8. The current sensitivity of a moving coil galvanometer increases by 20% when
its resistance is increased by a factor of two. Calculate by what factor, the
voltage sensitivity changes?
[3]
9. (a) Show how a moving coil galvanometer can be converted into an ammeter?
(b) A galvanometer has a resistance 30Ω and gives a full scale deflection for a
current of 2mA. How much resistance in what way must be connected to
convert into?
(1) An ammeter of range 0.3A
(2) A voltammeter of range 0.2V
=================
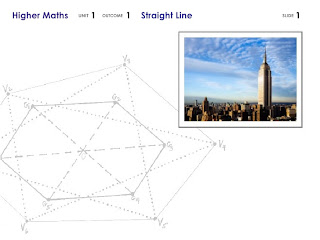
गणित में त्रिकोणमितीय फलनों के प्रतिलोम फलनों को प्रतिलोम त्रिकोणमितीय फलन (inverse trigonometric functions) कहते हैं। इनके डोमेन समुचित रूप से सीमित करके पारिभाषित किये गये हैं। इन्हें sin−1, cos−1 आदि के रूप में निरूपित करते हैं और 'साइन इन्वर्स' , 'कॉस इन्वर्स' आदि बोलते हैं।
होगा, यदि
होगा, यदि
होगा, यदि
होगा, यदि
होगा, यदि
होगा, यदि
उदाहरण:
अनुक्रम[छुपाएँ] |
[संपादित करें]मुख्य मान
चूँकि कोई भी त्रिकोणमितीय फलन एकैकी (one-to-one) नहीं है, इनके प्रतिलोम फलन तभी सम्भव होंगे यदि इनके डोमेन सीमित रखे जांय।
निम्नांकित सारणी में मुख्य प्रतिलोमों का विवरण दिया गया है-
नाम | सामान्य निरूपण | परिभाषा | वास्तविक परिणाम के लिये x का डोमेन | मुख्य मानों का परास (रेंज) (रेडियन) | मुख्य मानों का परास (डिग्री) |
---|---|---|---|---|---|
arcsine | y = arcsin x | x = sin y | −1 ≤ x ≤ 1 | −π/2 ≤ y ≤ π/2 | −90° ≤ y ≤ 90° |
arccosine | y = arccos x | x = cos y | −1 ≤ x ≤ 1 | 0 ≤ y ≤ π | 0° ≤ y ≤ 180° |
arctangent | y = arctan x | x = tan y | all real numbers | −π/2 < y < π/2 | −90° < y < 90° |
arccotangent | y = arccot x | x = cot y | all real numbers | 0 < y < π | 0° < y < 180° |
arcsecant | y = arcsec x | x = sec y | x ≤ −1 or 1 ≤ x | 0 ≤ y < π/2 or π/2 < y ≤ π | 0° ≤ y < 90° or 90° < y ≤ 180° |
arccosecant | y = arccsc x | x = csc y | x ≤ −1 or 1 ≤ x | −π/2 ≤ y < 0 or 0 < y ≤ π/2 | -90° ≤ y < 0° or 0° < y ≤ 90° |
यदि x को समिश्र संख्या होने की छूट हो तो y का रेंज केवल इसके वास्तविक भाग (real part) पर ही लागू होगा।
[संपादित करें]प्रतिलोम त्रिकोणमितीय फलनों में सम्बन्ध
Complementary angles:
Negative arguments:
Reciprocal arguments:
If you only have a fragment of a sine table:
Whenever the square root of a complex number is used here, we choose the root with the positive real part (or positive imaginary part if the square was negative real).
From the half-angle formula
, we get:
[संपादित करें]त्रिकोणमितीय फलनों एवं प्रतिलोम त्रिकोणमितीय फलनों में संबन्ध
[संपादित करें]सामान्य हल (General solutions)
निम्नलिखित में k कोई पूर्णांक है।
Determinants
For any square matrix of order 2, we have found a necessary and sufficient condition for invertibility. Indeed, consider the matrix
In order to generalize such notion to higher orders, we will need to study the determinant and see what kind of properties it satisfies. First let us use the following notation for the determinant
Properties of the Determinant
- 1.
- Any matrix A and its transpose have the same determinant, meaning
This is interesting since it implies that whenever we use rows, a similar behavior will result if we use columns. In particular we will see how row elementary operations are helpful in finding the determinant. Therefore, we have similar conclusions for elementary column operations. - 2.
- The determinant of a triangular matrix is the product of the entries on the diagonal, that is
- 3.
- If we interchange two rows, the determinant of the new matrix is the opposite of the old one, that is
- 4.
- If we multiply one row with a constant, the determinant of the new matrix is the determinant of the old one multiplied by the constant, that is
In particular, if all the entries in one row are zero, then the determinant is zero. - 5.
- If we add one row to another one multiplied by a constant, the determinant of the new matrix is the same as the old one, that is
Note that whenever you want to replace a row by something (through elementary operations), do not multiply the row itself by a constant. Otherwise, you will easily make errors (due to Property 4). - 6.
- We have
In particular, if A is invertible (which happens if and only if), then
If A and B are similar, then.
Let us look at an example, to see how these properties work.
Example. Evaluate
Determinants of Matrices of Higher Order
As we said before, the idea is to assume that previous properties satisfied by the determinant of matrices of order 2, are still valid in general. In other words, we assume:
- 1.
- Any matrix A and its transpose have the same determinant, meaning
- 2.
- The determinant of a triangular matrix is the product of the entries on the diagonal.
- 3.
- If we interchange two rows, the determinant of the new matrix is the opposite of the old one.
- 4.
- If we multiply one row with a constant, the determinant of the new matrix is the determinant of the old one multiplied by the constant.
- 5.
- If we add one row to another one multiplied by a constant, the determinant of the new matrix is the same as the old one.
- 6.
- We have
In particular, if A is invertible (which happens if and only if), then
So let us see how this works in case of a matrix of order 4.
Example. Evaluate
These calculations seem to be rather lengthy. We will see later on that a general formula for the determinant does exist.
Example. Evaluate
Example. Evaluate
General Formula for the Determinant Let A be a square matrix of order n. Write A = (aij), where aij is the entry on the row number i and the column number j, for
and
. For any i andj, set Aij (called the cofactors) to be the determinant of the square matrix of order (n-1) obtained from A by removing the row number i and the column number j multiplied by (-1)i+j. We have
In particular, we have along the rows
Example. Evaluate
Which technique to evaluate a determinant is easier ? The answer depends on the person who is evaluating the determinant. Some like the elementary row operations and some like the general formula. All that matters is to get the correct answer.
Note that all of the above properties are still valid in the general case. Also you should remember that the concept of a determinant only exists for square matrices.
Determinants: 2×2 Determinants
Determinants are like matrices, but done up in absolute-value bars instead of square brackets. There is a lot that you can do with (and learn from) determinants, but you'll need to wait for an advanced course to learn about them. In this lesson, I'll just show you how to compute 2×2 and 3×3 determinants. (It is possible to compute larger determinants, but the process is much more complicated.)
If you have a square matrix, its determinant is written by taking the same grid of numbers and putting them inside absolute-value bars instead of square brackets:
If this is "the matrix A" (or "A")...
|
...then this is "the determinant
of A" (or "det A"). |
Just as absolute values can be evaluated and simplified to get a single number, so can determinants. The process for evaluating determinants is pretty messy, so let's start simple, with the 2×2 case.
For a 2×2 matrix, its determinant is found by subtracting the products of its diagonals, which is a fancy way of saying in words what the following says in pictures:
the matrix A
|
the determinant of A ("det A")
|
the matrix A
|
the determinant of A ("det A")
|
In other words, to take the determinant of a 2×2 matrix, you multiply the top-left-to-bottom-right diagonal, and from this you subtract the product of bottom-left-to-top-right diagonal.
"But wait!" I hear you cry; "Aren't absolute values always supposed to be positive? You show that second matrix above as having a negative determinant. What's up with that?" You make a good point. Determinants are similar to absolute values, and use the same notation, but they are not identical, and one of the differences is that determinants can indeed be negative.
- Evaluate the following determinant:
- Find the determinant of the following matrix:
- I convert from a matrix to a determinant, multiply along the diagonals, subtract, and simplify:
Determinants: 3×3 Determinants
Sections: 2×2 determinants, 3×3 determinants
The computations for 3×3 determinants are messier than for 2×2's. Various methods can be used, but the simplest is probably the following: Copyright © Elizabeth Stapel 2004-2011 All Rights Reserved
The beginnings of matrices and determinants goes back to the second century BC although traces can be seen back to the fourth century BC. However it was not until near the end of the 17th Century that the ideas reappeared and development really got underway.
Our late 20th Century methods would have us write the linear equations as the rows of the matrix rather than the columns but of course the method is identical. Most remarkably the author, writing in 200 BC, instructs the reader to multiply the middle column by 3 and subtract the right column as many times as possible, the same is then done subtracting the right column as many times as possible from 3 times the first column. This gives
Next the left most column is multiplied by 5 and then the middle column is subtracted as many times as possible. This gives
from which the solution can be found for the third type of corn, then for the second, then the first by back substitution. This method, now known as Gaussian elimination, would not become well known until the early 19thCentury.
had a solution because
Addition or subtraction is accomplished by adding or subtracting corresponding elements. For example, consider matrix A and matrix B.
Both matrices have the same number of rows and columns (2 rows and 3 columns), so they can be added and subtracted. Thus,
And,
And finally, note that the order in which matrices are added is not important; thus, A + B = B + A.
Consider the matrices shown below - A, B, C, and D
Which of the following statements are true?
The correct answer is (C), as shown below.
Note that Matrices A and B cannot be added, because B has more columns than A. Matrices may be added or subtracted only if they have the same number of rows and the same number of columns.
=============
For example, if x is 5, and the matrix A is:
Then,
In the example above, every element of A is multiplied by 5 to produce the scalar multiple, B.
Note: Some texts refer to this operation as multiplying a matrix by a scalar. (A scalar is a real number or a symbol representing a real number.)
Suppose that A is an i x j matrix, and B is a j x k matrix. Then, the matrix product AB results in a matrix C, which has i rows and k columns; and each element in C can be computed according to the following formula.
Let AB = C. Because A has 2 rows, we know that C will have two rows; and because B has 2 columns, we know that C will have 2 columns. To compute the value of every element in the 2 x 2 matrix C, we use the formula Cik = Σj AijBjk, as shown below.
What we did to compute Matrix C was not complicated. All we did was to multiply row elements in Matrix A by corresponding column elements in Matrix B.
Because order is important, matrix algebra jargon has evolved to clearly indicate the order in which matrices are multiplied.
The identity matrix has a unique talent. Any matrix that can be premultiplied or postmultiplied by Iremains the same; that is:
Consider the matrices shown below - A, B, and C
Assume that AB = C. Which of the following statements are true?
The correct answer is (B). To compute the value of any element in matrix C, we use the formulaCik = Σj AijBjk.
In matrix C, x is the element in row 1 and column 2, which is represented in the formula by C12. Therefore, to find x, we use the formula to calculate C12, as shown below.
========
For example, suppose you want to interchange rows 1 and 2 of Matrix A. To accomplish this, you could premultiply A by E to produce B, as shown below.
Here, E is an elementary operator. It operates on A to produce the desired interchanged rows in B. What we would like to know, of course, is how to find E. Read on.
Then, to interchange the first and second columns of A, we postmultiply A by E, as shown below.
Note that the process for performing an elementary column operation on an r x c matrix is very similar to the process for performing an elementary row operation. The main differences are:
Assume that A is a 4 x 3 matrix. Suppose you want to multiply each element in the second column of matrix A by 9. Find the elementary column operator E.
Solution
To find the elementary column operator E, we multiply each element in the second column of the identity matrix I3 by 9.
Note: Some references present a slightly different description of the row echelon form. They do not require that the first non-zero entry in each row is equal to 1.
Which of the following matrices is in row echelon form?
The correct answer is (B), since it satisfies all of the requirements for a row echelon matrix. The other matrices fall short.
Which of the following matrices are in reduced row echelon form?
The correct answer is (D), since each matrix satisfies all of the requirements for a reduced row echelon matrix.
Matrix A is in row echelon form, and matrix B is in reduced row echelon form.
To transform matrix A into its echelon forms, we implemented the following series of elementary row operations.
Consider the matrix X, shown below.
Which of the following matrices is the reduced row echelon form of matrix X ?
The correct answer is (B). The elementary row operations used to change Matrix X into its reduced row echelon form are shown below.
To change X to its reduced row echelon form, we take the following steps:
For example, suppose a = 2b + 3c, as shown below.
Note that 2b is a scalar multiple and 3c is a scalar multiple. Thus, a is a linear combination of b andc.
Consider the row vectors below.
Note the following:
Consider the row vectors shown below.
Which of the following statements are true?
The correct answer is (D), as shown below.
Sections: 2×2 determinants, 3×3 determinants
The computations for 3×3 determinants are messier than for 2×2's. Various methods can be used, but the simplest is probably the following: Copyright © Elizabeth Stapel 2004-2011 All Rights Reserved
Take a matrix A: |
Write down its determinant: |
Extend the determinant's grid by rewriting the first two columns of numbers: |
Then multiply along the down-diagonals: |
...and along the up-diagonals |
Add the down-diagonals and subtract the up-diagonals: |
And simplify: |
- Then det(A) = 1.
- Find the deteriminant of the following matrix:
First I convert from the matrix to its determinant, with the extra columns: |
Then I multiply down and up the diagonals: |
The beginnings of matrices and determinants goes back to the second century BC although traces can be seen back to the fourth century BC. However it was not until near the end of the 17th Century that the ideas reappeared and development really got underway.
It is not surprising that the beginnings of matrices and determinants should arise through the study of systems of linear equations. The Babylonians studied problems which lead to simultaneous linear equations and some of these are preserved in clay tablets which survive. For example a tablet dating from around 300 BC contains the following problem:-
There are two fields whose total area is 1800 square yards. One produces grain at the rate of 2/3 of a bushel per square yard while the other produces grain at the rate of 1/2 a bushel per square yard. If the total yield is 1100 bushels, what is the size of each field.
The Chinese, between 200 BC and 100 BC, came much closer to matrices than the Babylonians. Indeed it is fair to say that the text Nine Chapters on the Mathematical Art written during the Han Dynasty gives the first known example of matrix methods. First a problem is set up which is similar to the Babylonian example given above:-
There are three types of corn, of which three bundles of the first, two of the second, and one of the third make 39 measures. Two of the first, three of the second and one of the third make 34 measures. And one of the first, two of the second and three of the third make 26 measures. How many measures of corn are contained of one bundle of each type?
Now the author does something quite remarkable. He sets up the coefficients of the system of three linear equations in three unknowns as a table on a 'counting board'.
1 2 3 2 3 2 3 1 1 26 34 39
Our late 20th Century methods would have us write the linear equations as the rows of the matrix rather than the columns but of course the method is identical. Most remarkably the author, writing in 200 BC, instructs the reader to multiply the middle column by 3 and subtract the right column as many times as possible, the same is then done subtracting the right column as many times as possible from 3 times the first column. This gives
0 0 3 4 5 2 8 1 1 39 24 39
Next the left most column is multiplied by 5 and then the middle column is subtracted as many times as possible. This gives
0 0 3 0 5 2 36 1 1 99 24 39
from which the solution can be found for the third type of corn, then for the second, then the first by back substitution. This method, now known as Gaussian elimination, would not become well known until the early 19thCentury.
Cardan, in Ars Magna (1545), gives a rule for solving a system of two linear equations which he calls regula de modo and which [7] calls mother of rules ! This rule gives what essentially is Cramer's rule for solving a 2
2 system although Cardan does not make the final step. Cardan therefore does not reach the definition of a determinant but, with the advantage of hindsight, we can see that his method does lead to the definition.
Many standard results of elementary matrix theory first appeared long before matrices were the object of mathematical investigation. For example de Witt in Elements of curves, published as a part of the commentaries on the 1660 Latin version of Descartes' Géométrie , showed how a transformation of the axes reduces a given equation for a conic to canonical form. This amounts to diagonalising a symmetric matrix but de Witt never thought in these terms.
The idea of a determinant appeared in Japan and Europe at almost exactly the same time although Seki in Japan certainly published first. In 1683 Seki wrote Method of solving the dissimulated problems which contains matrix methods written as tables in exactly the way the Chinese methods described above were constructed. Without having any word which corresponds to 'determinant' Seki still introduced determinants and gave general methods for calculating them based on examples. Using his 'determinants' Seki was able to find determinants of 2
2, 3
3, 4
4 and 5
5 matrices and applied them to solving equations but not systems of linear equations.
Rather remarkably the first appearance of a determinant in Europe appeared in exactly the same year 1683. In that year Leibniz wrote to de l'Hôpital. He explained that the system of equations
10 + 11x + 12y = 0 20 + 21x + 22y = 0 30 + 31x + 32y = 0
had a solution because
10.21.32 + 11.22.30 + 12.20.31 = 10.22.31 + 11.20.32 + 12.21.30
which is exactly the condition that the coefficient matrix has determinant 0. Notice that here Leibniz is not using numerical coefficients but
two characters, the first marking in which equation it occurs, the second marking which letter it belongs to.
Hence 21 denotes what we might write as a21.
Leibniz was convinced that good mathematical notation was the key to progress so he experimented with different notation for coefficient systems. His unpublished manuscripts contain more than 50 different ways of writing coefficient systems which he worked on during a period of 50 years beginning in 1678. Only two publications (1700 and 1710) contain results on coefficient systems and these use the same notation as in his letter tode l'Hôpital mentioned above.
Leibniz used the word 'resultant' for certain combinatorial sums of terms of a determinant. He proved various results on resultants including what is essentially Cramer's rule. He also knew that a determinant could be expanded using any column - what is now called the Laplace expansion. As well as studying coefficient systems of equations which led him to determinants, Leibniz also studied coefficient systems of quadratic forms which led naturally towards matrix theory.
In the 1730's Maclaurin wrote Treatise of algebra although it was not published until 1748, two years after his death. It contains the first published results on determinants proving Cramer's rule for 2
2 and 3
3 systems and indicating how the 4
4 case would work. Cramer gave the general rule for n
n systems in a paper Introduction to the analysis of algebraic curves (1750). It arose out of a desire to find the equation of a plane curve passing through a number of given points. The rule appears in an Appendix to the paper but no proof is given:-
One finds the value of each unknown by forming n fractions of which the common denominator has as many terms as there are permutations of n things.
Cramer does go on to explain precisely how one calculates these terms as products of certain coefficients in the equations and how one determines the sign. He also says how the n numerators of the fractions can be found by replacing certain coefficients in this calculation by constant terms of the system.
Work on determinants now began to appear regularly. In 1764 Bezout gave methods of calculating determinants as did Vandermonde in 1771. In 1772 Laplace claimed that the methods introduced by Cramer and Bezoutwere impractical and, in a paper where he studied the orbits of the inner planets, he discussed the solution of systems of linear equations without actually calculating it, by using determinants. Rather surprisingly Laplace used the word 'resultant' for what we now call the determinant: surprising since it is the same word as used by Leibniz yet Laplace must have been unaware of Leibniz's work. Laplace gave the expansion of a determinant which is now named after him.
Lagrange, in a paper of 1773, studied identities for 3
3 functional determinants. However this comment is made with hindsight since Lagrange himself saw no connection between his work and that of Laplace andVandermonde. This 1773 paper on mechanics, however, contains what we now think of as the volume interpretation of a determinant for the first time. Lagrange showed that the tetrahedron formed by O(0,0,0) and the three points M(x,y,z), M'(x',y',z'), M"(x",y",z") has volume
1/6 [z(x'y" - y'x") + z'(yx" - xy") + z"(xy' - yx')].
The term 'determinant' was first introduced by Gauss in Disquisitiones arithmeticae (1801) while discussing quadratic forms. He used the term because the determinant determines the properties of the quadratic form. However the concept is not the same as that of our determinant. In the same work Gauss lays out the coefficients of his quadratic forms in rectangular arrays. He describes matrix multiplication (which he thinks of as composition so he has not yet reached the concept of matrix algebra) and the inverse of a matrix in the particular context of the arrays of coefficients of quadratic forms.
Gaussian elimination, which first appeared in the text Nine Chapters on the Mathematical Art written in 200 BC, was used by Gauss in his work which studied the orbit of the asteroid Pallas. Using observations of Pallas taken between 1803 and 1809, Gauss obtained a system of six linear equations in six unknowns. Gauss gave a systematic method for solving such equations which is precisely Gaussian elimination on the coefficient matrix.
It was Cauchy in 1812 who used 'determinant' in its modern sense. Cauchy's work is the most complete of the early works on determinants. He reproved the earlier results and gave new results of his own on minors and adjoints. In the 1812 paper the multiplication theorem for determinants is proved for the first time although, at the same meeting of the Institut de France, Binet also read a paper which contained a proof of the multiplication theorem but it was less satisfactory than that given by Cauchy.
In 1826 Cauchy, in the context of quadratic forms in n variables, used the term 'tableau' for the matrix of coefficients. He found the eigenvalues and gave results on diagonalisation of a matrix in the context of converting a form to the sum of squares. Cauchy also introduced the idea of similar matrices (but not the term) and showed that if two matrices are similar they have the same characteristic equation. He also, again in the context of quadratic forms, proved that every real symmetric matrix is diagonalisable.
Jacques Sturm gave a generalisation of the eigenvalue problem in the context of solving systems of ordinary differential equations. In fact the concept of an eigenvalue appeared 80 years earlier, again in work on systems of linear differential equations, by D'Alembert studying the motion of a string with masses attached to it at various points.
It should be stressed that neither Cauchy nor Jacques Sturm realised the generality of the ideas they were introducing and saw them only in the specific contexts in which they were working. Jacobi from around 1830 and then Kronecker and Weierstrass in the 1850's and 1860's also looked at matrix results but again in a special context, this time the notion of a linear transformation. Jacobi published three treatises on determinants in 1841. These were important in that for the first time the definition of the determinant was made in an algorithmic way and the entries in the determinant were not specified so his results applied equally well to cases were the entries were numbers or to where they were functions. These three papers by Jacobi made the idea of a determinant widely known.
Cayley, also writing in 1841, published the first English contribution to the theory of determinants. In this paper he used two vertical lines on either side of the array to denote the determinant, a notation which has now become standard.
Eisenstein in 1844 denoted linear substitutions by a single letter and showed how to add and multiply them like ordinary numbers except for the lack of commutativity. It is fair to say that Eisenstein was the first to think of linear substitutions as forming an algebra as can be seen in this quote from his 1844 paper:-
An algorithm for calculation can be based on this, it consists of applying the usual rules for the operations of multiplication, division, and exponentiation to symbolic equations between linear systems, correct symbolic equations are always obtained, the sole consideration being that the order of the factors may not be altered.
The first to use the term 'matrix' was Sylvester in 1850. Sylvester defined a matrix to be an oblong arrangement of terms and saw it as something which led to various determinants from square arrays contained within it. After leaving America and returning to England in 1851, Sylvester became a lawyer and met Cayley, a fellow lawyer who shared his interest in mathematics. Cayley quickly saw the significance of the matrix concept and by 1853 Cayley had published a note giving, for the first time, the inverse of a matrix.
Cayley in 1858 published Memoir on the theory of matrices which is remarkable for containing the first abstract definition of a matrix. He shows that the coefficient arrays studied earlier for quadratic forms and for linear transformations are special cases of his general concept. Cayley gave a matrix algebra defining addition, multiplication, scalar multiplication and inverses. He gave an explicit construction of the inverse of a matrix in terms of the determinant of the matrix. Cayley also proved that, in the case of 2
2 matrices, that a matrix satisfies its own characteristic equation. He stated that he had checked the result for 3
3 matrices, indicating its proof, but says:-
I have not thought it necessary to undertake the labour of a formal proof of the theorem in the general case of a matrix of any degree.
That a matrix satisfies its own characteristic equation is called the Cayley-Hamilton theorem so its reasonable to ask what it has to do with Hamilton. In fact he also proved a special case of the theorem, the 4
4 case, in the course of his investigations into quaternions.
In 1870 the Jordan canonical form appeared in Treatise on substitutions and algebraic equations by Jordan. It appears in the context of a canonical form for linear substitutions over the finite field of order a prime.
Frobenius, in 1878, wrote an important work on matrices On linear substitutions and bilinear forms although he seemed unaware of Cayley's work. Frobenius in this paper deals with coefficients of forms and does not use the term matrix. However he proved important results on canonical matrices as representatives of equivalence classes of matrices. He cites Kronecker and Weierstrass as having considered special cases of his results in 1874 and 1868 respectively. Frobenius also proved the general result that a matrix satisfies its characteristic equation. This 1878 paper by Frobenius also contains the definition of the rank of a matrix which he used in his work on canonical forms and the definition of orthogonal matrices.
The nullity of a square matrix was defined by Sylvester in 1884. He defined the nullity of A, n(A), to be the largest i such that every minor of A of order n-i+1 is zero. Sylvester was interested in invariants of matrices, that is properties which are not changed by certain transformations. Sylvester proved that
max{n(A), n(B)} ≤ n(AB) ≤ n(A) + n(B).
In 1896 Frobenius became aware of Cayley's 1858 Memoir on the theory of matrices and after this started to use the term matrix. Despite the fact that Cayley only proved the Cayley-Hamilton theorem for 2
2 and 3
3 matrices, Frobenius generously attributed the result to Cayley despite the fact that Frobenius had been the first to prove the general theorem.
An axiomatic definition of a determinant was used by Weierstrass in his lectures and, after his death, it was published in 1903 in the note On determinant theory. In the same year Kronecker's lectures on determinants were also published, again after his death. With these two publications the modern theory of determinants was in place but matrix theory took slightly longer to become a fully accepted theory. An important early text which brought matrices into their proper place within mathematics was Introduction to higher algebra by Bôcher in 1907. Turnbull and Aitken wrote influential texts in the 1930's and Mirsky's An introduction to linear algebra in 1955 saw matrix theory reach its present major role in as one of the most important undergraduate mathematics topic.
References (13 books/articles)
Article by: J J O'Connor and E F Robertson
Cramer's Rule
Given a system of linear equations, Cramer's Rule is a handy way to solve for just one of the variables without having to solve the whole system of equations. They don't usually teach Cramer's Rule this way, but this is supposed to be the point of the Rule: instead of solving the entire system of equations, you can use Cramer's to solve for just one single variable.
Let's use the following system of equations:
- 2x + y + z = 3
x – y – z = 0
x + 2y + z = 0
We have the left-hand side of the system with the variables (the "coefficient matrix") and the right-hand side with the answer values. Let D be the determinant of the coefficient matrix of the above system, and let Dx be the determinant formed by replacing the x-column values with the answer-column values:
system of
equations |
coefficient
matrix's determinant |
answer
column |
Dx: coefficient determinant
with answer-column values in x-column |
2x + 1y + 1z = 3
1x – 1y – 1z = 0 1x + 2y + 1z = 0 |
Similarly, Dy and Dz would then be: Copyright © Elizabeth Stapel 2004-2011 All Rights Reserved
Evaluating each determinant, we get:
Cramer's Rule says that x = Dx ÷ D, y = Dy ÷ D, and z = Dz ÷ D. That is:
- x = 3/3 = 1, y = –6/3 = –2, and z = 9/3 = 3
That's all there is to Cramer's Rule. To find whichever variable you want (call it "ß" or "beta"), just evaluate the determinant quotient Dß ÷ D. (Please don't ask me to explain why this works. Just trust me that determinants can work many kinds of magic.)
- Given the following system of equations, find the value of z.
- 2x + y + z = 1
x – y + 4z = 0
x + 2y – 2z = 3
Then I form the quotient and simplify: |
- z = 2
The point of Cramer's Rule is that you don't have to solve the whole system to get the one value you need. This saved me a fair amount of time on some physics tests. I forget what we were working on (something with wires and currents, I think), but Cramer's Rule was so much faster than any other solution method (and God knows I needed the extra time). Don't let all the subscripts and stuff confuse you; the Rule is really pretty simple. You just pick the variable you want to solve for, replace that variable's column of values in the coefficient determinant with the answer-column's values, evaluate that determinant, and divide by the coefficient determinant. That's all there is to it.
Almost.
What if the coefficient determinant is zero? You can't divide by zero, so what does this mean? I can't go into the technicalities here, but "D = 0" means that the system of equations has no unique solution. The system may be inconsistent (no solution at all) or dependent (an infinite solution, which may be expressed as a parametric solution such as "(a, a + 3, a – 4)"). In terms of Cramer's Rule, "D = 0" means that you'll have to use some other method (such as matrix row operations) to solve the system. If D = 0, you can't use Cramer's Rule.
=============================================================================
Types of Matrices
This lesson describes a few of the more important types of matrices: transpose matrices, vectors, and different kinds of square matrices.
Transpose Matrix
The transpose of one matrix is another matrix that is obtained by using by using rows from the first matrix as columns in the second matrix.
For example, it is easy to see that the transpose of matrix A is A'. Row 1 of matrix A becomes column 1 of A'; row 2 of A becomes column 2 of A'; and row 3 of A becomes column 3 of A'.
|
|
Note that the order of a matrix is reversed after it has been transposed. Matrix A is a 3 x 2 matrix, but matrix A' is a 2 x 3 matrix.
With respect to notation, this web site uses a prime to indicate a transpose. Thus, the transpose of matrix B would be written as B'.
Vectors
Vectors are a type of matrix having only one column or one row.
Vectors come in two flavors: column vectors and row vectors. For example, matrix a is a column vector, and matrix a' is a row vector.
|
|
We use lower-case, boldface letters to represent column vectors. And since the transpose of a column vector is a row vector, we use lower-case, boldface letters plus a prime to represent row vectors. Thus, vector b would be a column vector, and vector b' would be a row vector.
Square Matrices
A square matrix is an n x n matrix; that is, a matrix with the same number of rows as columns. In this section, we describe several special kinds of square matrix.
- Symmetric matrix. If the transpose of a matrix is equal to itself, that matrix is said to be symmetric. Two examples of symmetric matrices appear below.
A = A' = 1 2 2 3 B = B' = 5 6 7 6 3 2 7 2 1 Note that each of these matrices satisfy the defining requirement of a symmetric matrix: A =A' and B = B'. - Diagonal matrix. A diagonal matrix is a special kind of symmetric matrix. It is a symmetric matrix with zeros in the off-diagonal elements. Two diagonal matrices are shown below.
A = 1 0 0 3 B = 5 0 0 0 3 0 0 0 1 Note that the diagonal of a matrix refers to the elements that run from the upper left corner to the lower right corner. - Scalar matrix. A scalar matrix is a special kind of diagonal matrix. It is a diagonal matrix with equal-valued elements along the diagonal. Two examples of a scalar matrix appear below.
A = 3 0 0 3 B = 5 0 0 0 5 0 0 0 5
These square matrices play a prominent role in the application of matrix algebra to real-world problems. For example, a scalar matrix called the identity matrix is critical to the solution of simultaneous linear equations. (We cover the identity matrix later in the tutorial.)
Test Your Understanding of This Lesson
Problem 1
Consider the matrices shown below - a, A, B, and C
|
|
|
|
Which of the following statements are true?
I. a is a row matrix
II. A = B'
III. C is a symmetric matrix
II. A = B'
III. C is a symmetric matrix
(A) I and II
(B) I and III
(C) II and III
(D) None of the above
(E) All of the above
(B) I and III
(C) II and III
(D) None of the above
(E) All of the above
Solution
The correct answer is (C), as explained below.
- Matrix a is a column vector, not a row matrix
- The transpose of a matrix is created by interchanging corresponding rows and columns. When this is done to matrix B, we see that A = B'.
A = 3 5 4 6 B = 3 4 5 6 B' = 3 5 4 6 so A = B' - The transpose of C is equal to C; that is C = C'. Therefore, C is a symmetric matrix.
Note that the off-diagonal elements of matrix C are equal to zero; so matrix C is a diagonal matrix, which is a special kind of symmetric matrix.
Matrix Addition and Matrix Subtraction
Just like ordinary algebra, matrix algebra has operations like addition and subtraction.How to Add and Subtract Matrices
Two matrices may be added or subtracted only if they have the same dimension; that is, they must have the same number of rows and columns.Addition or subtraction is accomplished by adding or subtracting corresponding elements. For example, consider matrix A and matrix B.
A = |
| B = |
|
A + B = |
| = |
|
A - B = |
| = |
|
Test Your Understanding of This Lesson
Problem 1Consider the matrices shown below - A, B, C, and D
|
|
|
|
I. A + B = C
II. B + C = D
III. B - C = D
II. B + C = D
III. B - C = D
(A) I only
(B) II only
(C) III only
(D) I and II
(E) I and III
Solution(B) II only
(C) III only
(D) I and II
(E) I and III
The correct answer is (C), as shown below.
|
=============
Matrix Multiplication
In matrix algebra, there are two kinds of matrix multiplication: multiplication of a matrix by a number and multiplication of a matrix by another matrix.How to Multiply a Matrix by a Number
When you multiply a matrix by a number, you multiply every element in the matrix by the same number. This operation produces a new matrix, which is called a scalar multiple.For example, if x is 5, and the matrix A is:
A = |
|
xA = 5A = 5 |
| = |
| = |
| = B |
Note: Some texts refer to this operation as multiplying a matrix by a scalar. (A scalar is a real number or a symbol representing a real number.)
How to Multiply a Matrix by a Matrix
The matrix product AB is defined only when the number of columns in A is equal to the number of rows in B. Similarly, the matrix product BA is defined only when the number of columns in B is equal to the number of rows in A.Suppose that A is an i x j matrix, and B is a j x k matrix. Then, the matrix product AB results in a matrix C, which has i rows and k columns; and each element in C can be computed according to the following formula.
Cik = Σj AijBjk
where
Cik = the element in row i and column k from matrix C
Aij = the element in row i and column j from matrix A
Bjk = the element in row j and column k from matrix B
Σj = summation sign, which indicates that the aijbjk terms should be summed over j
Let's work through an example to show how the above formula works. Suppose we want to computeAB, given the matrices below.Aij = the element in row i and column j from matrix A
Bjk = the element in row j and column k from matrix B
Σj = summation sign, which indicates that the aijbjk terms should be summed over j
A = |
| B = |
|
- C11 = Σ A1jBj1 = 0*6 + 1*8 +2*10 = 0 + 8 + 20 = 28
- C12 = Σ A1jBj2 = 0*7 + 1*9 +2*11 = 0 + 9 + 22 = 31
- C21 = Σ A2jBj1 = 3*6 + 4*8 +5*10 = = 18 + 32 + 50 = 100
- C22 = Σ A2jBj2 = 3*7 + 4*9 +5*11 = 21 + 36 +55 = 112
AB = C = |
|
Multiplication Order
As we have already mentioned, in some cases, matrix multiplication is defined for AB, but not forBA; and vice versa. However, even when matrix multiplication is possible in both directions, results may be different. That is, AB is not always equal to BA.Because order is important, matrix algebra jargon has evolved to clearly indicate the order in which matrices are multiplied.
- To describe the matrix product AB, we can say A is postmultiplied by B; or we can say that B ispremultiplied by A.
- Similarly, to describe the matrix product BA, we can say B is postmultiplied by A; or we can say that A is premultiplied by B.
Identity Matrix
The identity matrix is an n x n diagonal matrix with 1's in the diagonal and zeros everywhere else. The identity matrix is denoted by I or In. Two identity matrices appear below.
|
|
AI = IA = A
Test Your Understanding of This Lesson
Problem 1Consider the matrices shown below - A, B, and C
|
|
|
(A) w = a*e + b*h
(B) x = a*f + b*h
(C) y = c*g + d*h
(D) All of the above
(E) None of the above
Solution(B) x = a*f + b*h
(C) y = c*g + d*h
(D) All of the above
(E) None of the above
The correct answer is (B). To compute the value of any element in matrix C, we use the formulaCik = Σj AijBjk.
In matrix C, x is the element in row 1 and column 2, which is represented in the formula by C12. Therefore, to find x, we use the formula to calculate C12, as shown below.
x = C12 = Σj A1jBj2 = A11B12 + A12B22 = a*f + b*h
All of the other answers are incorrect.========
Elementary Matrix Operations
Elementary matrix operations play an important role in many matrix algebra applications, such asfinding the inverse of a matrix and solving simultaneous linear equations.Elementary Operations
There are three kinds of elementary matrix operations.- Interchange two rows (or columns).
- Multiply each element in a row (or column) by a non-zero number.
- Multiply a row (or column) by a non-zero number and add the result to another row (or column).
Elementary Operation Notation
In many references, you will encounter a compact notation to describe elementary operations. That notation is shown below.Operation description | Notation | |
---|---|---|
Row operations | 1. Interchange rows i and j | Ri <--> Rj |
2. Multiply row i by s, where s ≠ 0 | sRi --> Ri | |
3. Add s times row i to row j | sRi + Rj --> Rj | |
Column operations | 1. Interchange columns i and j | Ci <--> Cj |
2. Multiply column i by s, where s ≠ 0 | sCi --> Ci | |
3. Add s times column i to column j | sCi + Cj --> Cj |
Elementary Operators
Each type of elementary operation may be performed by matrix multiplication, using square matrices called elementary operators.For example, suppose you want to interchange rows 1 and 2 of Matrix A. To accomplish this, you could premultiply A by E to produce B, as shown below.
R1 <--> R2 = |
|
| = |
| = |
| |||||||||||||||||||||||||||||||
E | A | B |
How to Perform Elementary Row Operations
To perform an elementary row operation on a A, an r x c matrix, take the following steps.- To find E, the elementary row operator, apply the operation to an r x r identity matrix.
- To carry out the elementary row operation, premultiply A by E.
- Interchange two rows. Suppose we want to interchange the second and third rows of A, a 3 x 2 matrix. To create the elementary row operator E, we interchange the second and third rows of the identity matrix I3.
1 0 0 0 1 0 0 0 1 ⇒ 1 0 0 0 0 1 0 1 0 I3 E Then, to interchange the second and third rows of A, we premultiply A by E, as shown below.R2 <--> R3 = 1 0 0 0 0 1 0 1 0 0 1 2 3 4 5 = 1*0 + 0*2 + 0*4 1*1 + 0*3 + 0*5 0*0 + 0*2 + 1*4 0*1 + 0*3 + 1*5 0*0 + 1*2 + 0*4 0*1 + 1*3 + 0*5 = 0 1 4 5 2 3 E A B - Multiply a row by a number. Suppose we want to multiply each element in the second row of Matrix A by 7. Assume A is a 2 x 3 matrix. To create the elementary row operator E, we multiply each element in the second row of the identity matrix I2 by 7.
1 0 0 1 ⇒ 1 0 0 7 I2 E Then, to multiply each element in the second row of A by 7, we premultiply A by E.7R2 --> R2 = 1 0 0 7 0 1 2 3 4 5 = 1*0 + 0*3 1*1 + 0*4 1*2 + 0*5 0*0 + 7*3 0*1 + 7*4 0*2 + 7*5 = 0 1 2 21 28 35 E A B - Multiply a row and add it to another row. Assume A is a 2 x 2 matrix. Suppose we want to multiply each element in the first row of A by 3; and we want to add that result to the second row of A. For this operation, creating the elementary row operator is a two-step process. First, we multiply each element in the first row of the identity matrix I2 by 3. Next, we add the result of that multiplication to the second row of I2 to produce E.
1 0 0 1 ⇒ 1 0 0 + 3*1 1 + 3*0 ⇒ 1 0 3 1 I2 E Then, to multiply each element in the first row of A by 3 and add that result to the second row, we premultiply A by E2.3R1 + R2 --> R2 = 1 0 3 1 0 1 2 3 = 1*0 + 0*2 1*1 + 0*3 3*0 + 1*2 3*1 + 1*3 = 0 1 2 6 E A B
How to Perform Elementary Column Operations
To perform an elementary column operation on A, an r x c matrix, take the following steps.- To find E, the elementary column operator, apply the operation to an c x c identity matrix.
- To carry out the elementary column operation, postmultiply A by E.
| ⇒ |
| ||||||||||||
I2 | E |
C1 <--> C2 = |
|
| = |
| = |
| |||||||||||||||||||||||||||||||
A | E | B |
- To operate on the r x c matrix A, the row operator E is created from an r x r identity matrix; whereas the column operator E is created from an c x c identity matrix.
- To perform a row operation, A is premultiplied by E; whereas to perform a column operation,A is postmultiplied by E.
Test Your Understanding of This Lesson
Problem 1Assume that A is a 4 x 3 matrix. Suppose you want to multiply each element in the second column of matrix A by 9. Find the elementary column operator E.
Solution
To find the elementary column operator E, we multiply each element in the second column of the identity matrix I3 by 9.
| ⇒ |
| ||||||||||||||||||||||
I3 | E |
Echelon Form of a Matrix
This lesson introduces the concept of an echelon matrix. Echelon matrices come in two forms: therow echelon form (ref) and the reduced row echelon form (rref).Row Echelon Form
A matrix is in row echelon form (ref) when it satisfies the following conditions.- The first non-zero element in each row, called the leading entry, is 1.
- Each leading entry is in a column to the right of the leading entry in the previous row.
- Rows with all zero elements, if any, are below rows having a non-zero element.
|
|
| ||||||||||||||||||||||||||||||||||||||||||
Aref | Bref | Cref |
Reduced Row Echelon Form
A matrix is in reduced row echelon form (rref) when it satisfies the following conditions.- The matrix satisfies conditions for a row echelon form.
- The leading entry in each row is the only non-zero entry in its column.
|
|
| ||||||||||||||||||||||||||||||||||||||||||
Arref | Brref | Crref |
Test Your Understanding of This Lesson
Problem 1Which of the following matrices is in row echelon form?
|
|
|
| ||||||||||||||||||||||||||||||||
A | B | C | D |
(A) Matrix A
(B) Matrix B
(C) Matrix C
(D) Matrix D
(E) None of the above
Solution(B) Matrix B
(C) Matrix C
(D) Matrix D
(E) None of the above
The correct answer is (B), since it satisfies all of the requirements for a row echelon matrix. The other matrices fall short.
- The leading entry in Row 1 of matrix A is to the right of the leading entry in Row 2, which is inconsistent with definition of a row echelon matrix.
- In matrix C, the leading entries in Rows 2 and 3 are in the same column, which is not allowed.
- In matrix D, the row with all zeros (Row 2) comes before a row with a non-zero entry. This is a no-no.
Which of the following matrices are in reduced row echelon form?
|
|
| ||||||||||||||||||||||||||||||||||||||||||||||||||||||
A | B | C |
(A) Only matrix A
(B) Only matrix B
(C) Only matrix C
(D) All of the above
(E) None of the above
Solution(B) Only matrix B
(C) Only matrix C
(D) All of the above
(E) None of the above
The correct answer is (D), since each matrix satisfies all of the requirements for a reduced row echelon matrix.
- The first non-zero element in each row, called the leading entry, is 1.
- Each leading entry is in a column to the right of the leading entry in the previous row.
- Rows with all zero elements, if any, are below rows having a non-zero element.
- The leading entry in each row is the only non-zero entry in its column.
How to Change a Matrix Into its Echelon Form
This lesson shows how to convert a matrix to its row echelon form and to its reduced row echelon form.Echelon Forms
A matrix is in row echelon form (ref) when it satisfies the following conditions.- The first non-zero element in each row, called the leading entry, is 1.
- Each leading entry is in a column to the right of the leading entry in the previous row.
- Rows with all zero elements, if any, are below rows having a non-zero element.
- The matrix is in row echelon form (i.e., it satisfies the three conditions listed above).
- The leading entry in each row is the only non-zero entry in its column.
|
| |||||||||||||||||||||||||||||||||||||
A | B |
How to Transform a Matrix Into Its Echelon Forms
Any matrix can be transformed into its echelon forms, using a series of elementary row operations. Here's how.- Pivot the matrix
- Find the pivot, the first non-zero entry in the first column of the matrix.
- Interchange rows, moving the pivot row to the first row.
- Multiply each element in the pivot row by the inverse of the pivot, so the pivot equals 1.
- Add multiples of the pivot row to each of the lower rows, so every element in the pivot column of the lower rows equals 0.
- To get the matrix in row echelon form, repeat the pivot
- Repeat the procedure from Step 1 above, ignoring previous pivot rows.
- Continue until there are no more pivots to be processed.
- To get the matrix in reduced row echelon form, process non-zero entries above each pivot.
- Identify the last row having a pivot equal to 1, and let this be the pivot row.
- Add multiples of the pivot row to each of the upper rows, until every element above the pivot equals 0.
- Moving up the matrix, repeat this process for each row.
Transforming a Matrix Into Its Echelon Forms: An Example
To illustrate the transformation process, let's transform Matrix A to a row echelon form and to a reduced row echelon form.
| ⇒ |
| ⇒ |
| ⇒ |
| ⇒ |
| |||||||||||||||||||||||||||||||||||||||||||||||||||||||
A | A1 | A2 | Aref | Arref |
- We found the first non-zero entry in the first column of the matrix in row 2; so we interchanged Rows 1 and 2, resulting in matrix A1.
- Working with matrix A1, we multiplied each element of Row 1 by -2 and added the result to Row 3. This produced A2.
- Working with matrix A2, we multiplied each element of Row 2 by -3 and added the result to Row 3. This produced Aref. Notice that Aref is in row echelon form, because it meets the following requirements: (a) the first non-zero entry of each row is 1, (b) the first non-zero entry is to the right of the first non-zero entry in the previous row, and (c) rows made up entirely of zeros are at the bottom of the matrix.
- And finally, working with matrix Aref, we multiplied the second row by -2 and added it to the first row. This produced Arref. Notice that Arref is in reduced row echelon form, because it satisfies the requirements for row echelon form plus each leading non-zero entry is the only non-zero entry in its column.
Test Your Understanding of This Lesson
Problem 1Consider the matrix X, shown below.
X = |
|
|
|
|
| ||||||||||||||||||||||||||||||||
A | B | C | D |
(A) Matrix A
(B) Matrix B
(C) Matrix C
(D) Matrix D
(E) None of the above
Solution(B) Matrix B
(C) Matrix C
(D) Matrix D
(E) None of the above
The correct answer is (B). The elementary row operations used to change Matrix X into its reduced row echelon form are shown below.
| ⇒ |
| ⇒ |
| ⇒ |
| ||||||||||||||||||||||||||||||||
X | X1 | X2 | Xrref |
- Interchange Rows 1 and 2, producing X1.
- In X1, multiply Row 2 by -5 and add it to Row 3, producing X2.
- In X2, multiply Row 2 by -2 and add it to Row 1, producing Xrref.
Independent vs. Dependent Vectors
One vector is dependent on other vectors, if it is a linear combination of the other vectors.Linear Combination of Vectors
If one vector is equal to the sum of scalar multiples of other vectors, it is said to be a linear combination of the other vectors.For example, suppose a = 2b + 3c, as shown below.
| = | 2 |
| + | 3 |
| = |
| ||||||||||||||||
a | b | c |
Linear Dependence of Vectors
A set of vectors is linearly independent if no vector in the set is (a) a scalar multiple of another vector in the set or (b) a linear combination of other vectors in the set; conversely, a set of vectors is linearly dependent if any vector in the set is (a) a scalar multiple of another vector in the set or (b) a linear combination of other vectors in the set.Consider the row vectors below.
a = |
| d = |
| |||||||||||
b = |
| e = |
| |||||||||||
c = |
| f = |
|
- Vectors a and b are linearly independent, because neither vector is a scalar multiple of the other.
- Vectors a and d are linearly dependent, because d is a scalar multiple of a; i.e., d = 2a.
- Vector c is a linear combination of vectors a and b, because c = a + b. Therefore, the set of vectors a, b, and c is linearly dependent.
- Vectors d, e, and f are linearly independent, since no vector in the set can be derived as a scalar multiple or a linear combination of any other vectors in the set.
Test Your Understanding of This Lesson
Problem 1Consider the row vectors shown below.
|
|
|
| ||||||||||||||||||||
a | b | c | d |
(A) Vectors a, b, and c are linearly dependent.
(B) Vectors a, b, and d are linearly dependent.
(C) Vectors b, c, and d are linearly dependent.
(D) All of the above.
(E) None of the above.
Solution(B) Vectors a, b, and d are linearly dependent.
(C) Vectors b, c, and d are linearly dependent.
(D) All of the above.
(E) None of the above.
The correct answer is (D), as shown below.
- Vectors a, b, and c are linearly dependent, since a + b = c.
- Vectors a, b, and d are linearly dependent, since 2a + b = d.
- Vectors b, c, and d are linearly dependent, since 2c - b = d.
Matrix Rank
This lesson introduces the concept of matrix rank and explains how the rank of a matrix is revealed by its echelon form.
The Rank of a Matrix
You can think of an r x c matrix as a set of r row vectors, each having c elements; or you can think of it as a set of c column vectors, each having r elements.
The rank of a matrix is defined as (a) the maximum number of linearly independent column vectors in the matrix or (b) the maximum number of linearly independent row vectors in the matrix. Both definitions are equivalent.
For an r x c matrix,
- If r is less than c, then the maximum rank of the matrix is r.
- If r is greater than c, then the maximum rank of the matrix is c.
How to Find Matrix Rank
In this section, we describe a method for finding the rank of any matrix. This method assumes familiarity with echelon matrices and echelon transformations.
The maximum number of linearly independent vectors in a matrix is equal to the number of non-zero rows in its row echelon matrix. Therefore, to find the rank of a matrix, we simply transform the matrix to its row echelon form and count the number of non-zero rows.
Consider matrix A and its row echelon matrix, Aref. Previously, we showed how to find the row echelon form for matrix A.
0 1 2 1 2 1 2 7 8 ⇒ 1 2 1 0 1 2 0 0 0 A Aref
You can verify that this is correct. Row 1 and Row 2 of matrix A are linearly independent. However, Row 3 is a linear combination of Rows 1 and 2. Specifically, Row 3 = 3*( Row 1 ) + 2*( Row 2). Therefore, matrix A has only two independent row vectors.
Full Rank Matrices
When all of the vectors in a matrix are linearly independent, the matrix is said to be full rank. Consider the matrices A and B below.
A = 1 2 3 2 4 6 B = 1 0 2 2 1 0 3 2 1
Now, look at matrix B. All of its rows are linearly independent, so the rank of matrix B is 3. Matrix Bis full rank.
Test Your Understanding of This Lesson
Problem 1
Consider the matrix X, shown below.
X = 1 2 4 4 3 4 8 0
(A) 0Solution
(B) 1
(C) 2
(D) 3
(E) 4
The correct answer is (C). Since the matrix has more than zero elements, its rank must be greater than zero. And since it has fewer rows than columns, its maximum rank is equal to the maximum number of linearly independent rows. And because neither row is linearly dependent on the other row, the matrix has 2 linearly independent rows; so its rank is 2.
Problem 2
Consider the matrix Y, shown below.
Y = 1 2 3 2 3 5 3 4 7 4 5 9
(A) 0Solution
(B) 1
(C) 2
(D) 3
(E) 4
The correct answer is (C). Since the matrix has more than zero elements, its rank must be greater than zero. And since it has fewer columns than rows, its maximum rank is equal to the maximum number of linearly independent columns.
Columns 1 and 2 are independent, because neither can be derived as a scalar multiple of the other. However, column 3 is linearly dependent on columns 1 and 2, because column 3 is equal to column 1 plus column 2. That leaves the matrix with a maximum of two linearly independent columns; e.g., column 1 and column 2. So the matrix rank is 2.
Matrix Determinants
The determinant is a unique number associated with a square matrix. In this lesson, we show how to compute the determinant for any square matrix, and we introduce notation for matrix determinants.
Notation for a Determinant
There are at least three ways to denote the determinant of a square matrix.
- Denote the determinant by vertical lines around the matrix name; thus, the determinant of matrix A would be indicated by |A|.
- Another approach is to enclose matrix elements within vertical straight lines, as shown below.
|A| = A11 A12 A13 A21 A22 A23 A31 A32 A33 - And finally, some references refer to the deteriminant of A as Det A. Thus, |A| = Det A.
How to Compute the Determinant of a 2 x 2 Matrix
Suppose A is a 2 x 2 matrix with elements Aij, as shown below.
A = A11 A12 A21 A22
We compute the determinant of A according to the following formula.
|A| = ( A11 * A22 ) - ( A12 * A21 )How to Compute the Determinant of an n x n Matrix
The formula for computing the determinant of a 2 x 2 matrix (shown above) is actually a special case of the general algorithm for computing the determinant of any square matrix.
|A| = Σ ( + ) A1qA2rA3s . . . AnzThis algorithm requires some explanation. Here are the key points.
- The determinant is the sum of product terms made up of elements from the matrix.
- Each product term consists of n elements from the matrix.
- Each product term includes one element from each row and one element from each column.
- The number of product terms is equal to n! (where n! refers to n factorial).
- By convention, the elements of each product term are arranged in ascending order of the left-hand (or row-designating) subscript.
- To find the sign of each product term, we count the number of inversions needed to put the right-hand (or column-designating) subscripts in numerical order. If the number of inversions is even, the sign is positive; if odd, the sign is negative.
A = A11 A12 A13 A21 A22 A23 A31 A32 A33
|A| = Σ ( + ) A1qA2rA3s . . . AnzNote that we have 3! or 6 product terms, each consisting of one element from each row and one element from each column. The task remaining is to find the sign for each product term. To do this, we count the number of inversions needed to put elements in ascending order of their column-designating subscript.
|A| = + A11A22A33 + A12A23A31 + A13A21A32 + A13A22A31 + A12A21A33 + A11A23A32
To demonstrate how to count inversions, let's look at two of the product terms.
- Consider the second product term in the list: A12A23A31. To put all of the column-designating subscripts in ascending order, we need to move A31 from the end of the term to the front of the term, which results in: A31A12A23. This movement counts as two inversions, since we moved A31 two positions to the left. Since two is an even number, the sign of that product term should be positive.
- Consider the last product term in the list: A11A23A32. To put all of the column-designating subscripts in ascending order, we need to interchange the second and third elements, which results in: A11A32A23. This counts as one inversion, since we moved A32 one position to the left. Since one is an odd number, the sign of that product term should be negative.
|A| = Σ ( + ) A1qA2rA3s . . . AnzWe can employ the same process to compute the determinant for any size matrix. However, as the matrix gets larger, the number of product terms increases very quickly. For example, a 4 x 4 matrix would have 4! or 24 terms; a 5 x 5 matrix, 120 terms; a 6 x 6 matrix, 720 terms, and so on. A 10 x 10 matrix would have 3,628,800 terms. You would not want to calculate the determinant of a large matrix by hand.
|A| = + A11A22A33 + A12A23A31 + A13A21A32 - A13A22A31 - A12A21A33 - A11A23A32
Test Your Understanding of This Lesson
Problem 1
What is the determinant of matrix A?
A = 5 1 2 6 (A) -7Solution
(B) -28
(C) 7
(D) 28
(E) None of the above
The correct answer is (D), based on the matrix determinant algorithm shown below.
|A| = Σ ( + ) A1qA2rA3s . . . AnzBecause A is a 2 x 2 matrix, we know that the determinant algorithm has 2! or 2 product terms. And each product term includes one element from each row and one element from each column. We list the product terms below, with the elements of each product term arranged in ascending order of the left-hand (or row-designating) subscript.
|A| = + A11A22 + A12A21To determine whether each product term is preceded by a plus or minus sign, we count the inversions needed to put all of the column-designating subscripts in ascending order.
- The column-designating subscripts for the first term, A11A22, are already in ascending order; so the first term needs zero inversions. Since zero is an even number, the sign of the first term is positive.
- For the second term, A12a21, we must move the second element A21 one position to the left; that is, we need one inversion to put the column-designating subscripts in ascending order. Since one is an odd number, the sign of the second term is negative.
|A| = + A11A22 - A12A21So the determinant of matrix A is:
|A| = ( 5 * 6 ) - ( 1 * 2 ) = 30 - 2 = 28Matrix Inverse
This lesson defines the matrix inverse, and shows how to determine whether the inverse of a matrixexists.
Matrix Inversion
Suppose A is an n x n matrix. The inverse of A is another n x n matrix, denoted A-1, that satisfies the following conditions.
AA-1 = A-1A = Inwhere In is the identity matrix. Below, with an example, we illustrate the relationship between a matrix and its inverse.
2 1 3 4 0.8 -0.2 -0.6 0.4 = 0.8 -0.2 -0.6 0.4 2 1 3 4 = 1 0 0 1 A A-1 A-1 A I
Does the Inverse Exist?
There are two ways to determine whether the inverse of a square matrix exists.
- Determine its rank. The rank of a matrix is a unique number associated with a square matrix. If the rank of an n x n matrix is less than n, the matrix does not have an inverse. We showedhow to determine matrix rank previously.
- Compute its determinant. The determinant is another unique number associated with a square matrix. When the determinant for a square matrix is equal to zero, the inverse for that matrix does not exist. We showed how to find the determinant of a matrix previously.
Test Your Understanding of This Lesson
Problem 1
Consider the matrix A, shown below.
A = 2 4 1 2
(A) The rank of matrix A is 1.Solution
(B) The determinant of matrix A is 0.
(C) Matrix A is singular.
(D) All of the above.
(E) None of the above.
The correct answer is (D).
- The rank of a matrix is defined as the maximum number of linearly independent row vectors in the matrix. Rows 1 and 2 of matrix A are not independent, since Row 1 = 2 * Row 2. Therefore, A has only one independent row, so its rank is 1. Previously, we described how to compute matrix rank.
- Previously, we showed how to find the determinant of a 2 x 2 matrix. We use that approach to find the determinant of A, which is denoted |A|.|A| = ( A11 * A22 ) - ( A12 * A21 )
|A| = ( 2 * 2 ) - ( 4 * 1 ) = 4 - 4 = 0 - Matrix A is not a full rank matrix. And its determinant is equal to zero. Therefore, matrix Adoes not have an inverse, which means that matrix A is singular.
How to Find the Inverse of a Matrix: Special Cases
In this lesson, we show how to find the inverse of a matrix for two special cases: a diagonal matrixand a 2 x 2 matrix. In the next lesson, we show how to find the inverse for any matrix.
How to Find the Inverse of a Diagonal Matrix
A diagonal matrix matrix is a special kind of symmetric matrix. It is a symmetric matrix with zeros in the off-diagonal elements. Two diagonal matrices are shown below.
A = 1 0 0 3 B = 5 0 0 0 3 0 0 0 1
The inverse of a diagonal matrix is obtained by replacing each element in the diagonal with its reciprocal, as illustrated below for matrix C.
C = 2 0 0 4 C-1 = 1/2 0 0 1/4
CC-1 = C-1C = Iwhere I is the identity matrix.
This approach will work for any diagonal matrix, as long as none of the diagonal elements is equal to zero. If any of the diagonal elements are equal to zero, the matrix will be less than full rank, and the matrix will not have an inverse.
How to Find the Inverse of a 2 x 2 Matrix
Suppose A is a nonsingular matrix 2 x 2 matrix. Then, the inverse of A can be computed from A, as shown below.
A11 A12 A21 A22 A22/|A| -A12/|A| -A21/|A| A11/|A| A A-1
To illustrate how this works, let's find the inverse of matrix B, which appears below.
B = 2 1 4 4
|B| = B11B22 - B12B21 = 2*4 - 1*4 = 8 - 4 = 4Then, we can find the inverse, as shown below.
B-1 = B22/|B| -B12/|B| -B21/|B| B11/|B| = 4/4 -1/4 -4/4 2/4 = 1 -1/4 -1 1/2
Test Your Understanding of This Lesson
Problem 1
Find the inverse of matrix A, shown below.
A = 2 0 0 0
This was sort of a trick question. Matrix A is a diagonal matrix with a zero element in its diagonal. Therefore, matrix A is singular, and does not have an inverse.
Find the inverse of matrix A, shown below.
A = 2 0 0 8
The inverse of a diagonal matrix is obtained by replacing each element in the diagonal with its reciprocal, as shown below.
A-1 = 1/2 0 0 1/8
Find the inverse of matrix A, shown below.
A = 3 1 9 4
First, let's compute the determinant of matrix A.
|A| = A11A22 - A12A21 = 3*4 - 1*9 = 12 - 9 = 3Then, we can find the inverse, as shown below.
A-1 = A22/|A| -A12/|A| -A21/|A| A11/|A| = 4/3 -1/3 -9/3 3/3 = 4/3 -1/3 -3 1
How to Find the Inverse for Any Square Matrix
In this lesson, we describe a method for finding the inverse of any square matrix; and we demonstrate the method step-by-step with examples.
Prerequisites: This material assumes familiarity with elementary matrix operations and echelon transformations.
How to Find the Inverse of an n x n Matrix
Let A be an n x n matrix. To find the inverse of matrix A, we follow these steps:
- Using elementary operators, transform matrix A to its reduced row echelon form, Arref.
- Inspect Arref to determine if matrix A has an inverse.
- If Arref is equal to the identity matrix, then matrix A is full rank; and matrix A has an inverse.
- If the last row of Arref is all zeros, then matrix A is not full rank; and matrix A does not have an inverse.
- If A is full rank, then the inverse of matrix A is equal to the product of the elementary operators that produced Arref , as shown below.
A-1 = Er Er-1 . . . E2 E1whereA-1 = inverse of matrix ANote that the order in which elementary row operators are multiplied is important, because Ei Ej is not necessarily equal to Ej Ei.
r = Number of elementary row operations required to transform A to Arref
Ei = ith elementary row operator used to transform A to Arref
An Example of Finding the Inverse
Let's use the above method to find the inverse of matrix A, shown below.
A = 1 2 2 2 2 2 2 2 1
Elementary row operation Row operator, Ei Transformed matrix, Ai 1. Multiply row 1 of A by -2 and add the result to row 2 of AE1 = 1 0 0 -2 1 0 0 0 1 A1 = E1A = 1 2 2 0 -2 -2 2 2 1 2. Multiply row 1 of A1 by -2 and add the result to row 3 of A1E2 = 1 0 0 0 1 0 -2 0 1 A2 = E2A1 = 1 2 2 0 -2 -2 0 -2 -3 3. Multiply row 3 of A2 by -1 and add row 2 of A2 to row 3 of A2E3 = 1 0 0 0 1 0 0 1 -1 A3 = E3A2 = 1 2 2 0 -2 -2 0 0 1 4. Add row 2 of A3 to row 1 of A3E4 = 1 1 0 0 1 0 0 0 1 A4 = E4A3 = 1 0 0 0 -2 -2 0 0 1 5. Multiply row 2 of A4 by -0.5E5 = 1 0 0 0 -0.5 0 0 0 1 A5 = E5A4 = 1 0 0 0 1 1 0 0 1 6. Multiply row 3 of A5 by -1 and add the result to row 2 of A5E6 = 1 0 0 0 1 -1 0 0 1 Arref = E6A5 = 1 0 0 0 1 0 0 0 1
The last matrix in Step 6 of the above table is Arref, the reduced row echelon form for matrix A. Since Arref is equal to the identity matrix, we know that A is full rank. And because A is full rank, we know that A has an inverse.
If A were less than full rank, Arref would have all zeros in the last row; and A would not have an inverse.
We find the inverse of matrix A by computing the product of the elementary operators that producedArref , as shown below.
A-1 = E6 E5 E4 E3 E2 E1A-1 = 1 0 0 0 1 -1 0 0 1 1 0 0 0 -0.5 0 0 0 1 1 1 0 0 1 0 0 0 1 1 0 0 0 1 0 0 1 -1 1 0 0 0 1 0 -2 0 1 1 0 0 -2 1 0 0 0 1 E6 E5 E4 E3 E2 E1 A-1 = -1 1 0 1 -1.5 1 0 1 -1
Test Your Understanding of This Lesson
Problem
Find the inverse of matrix A, shown below.
A = 1 0 2 2
The first step is to transform matrix A into its reduced row echelon form, Arref, using elementary row operators Ei to perform elementary row operations, as shown below.
Elementary row operation Row operator, Ei Transformed matrix, Ai 1. Multiply row 1 of A by -2 and add the result to row 2 of AE1 = 1 0 -2 1 A1 = E1A = 1 0 0 2 2. Multiply row 2 of A1 by 0.5.E2 = 1 0 0 0.5 Arref = E2A1 = 1 0 0 1
We find the inverse by computing the product of the elementary operators that produced Arref , as shown below.
A-1 = E2 E1 = 1 0 0 0.5 1 0 -2 1 = 1 0 -1 0.5 E2 E1
No comments:
Post a Comment